Explore the future of Learning and Development technology trends with our exploration of data-driven learning analytics. Uncover insights into its evolution, current applications, and the transformative impact on learner engagement. Discover key components, benefits, and challenges, along with future trends shaping the L&D landscape. Dive into case studies, best practices, and ethical considerations, gaining a holistic perspective on the role of data-driven analytics in shaping the future of workplace learning.
Here’s a striking observation: Over half of organizations (56%) underscore data’s pivotal role in shaping business strategy. However, a paradox emerges—despite this acknowledgment, 58% of business stakeholders resist integrating data into their key decision-making processes.
Now, let’s pivot this statistic to the realm of L&D technology, where data-driven insights are poised to reshape the future of education. In a landscape where numbers are increasingly recognized as the compass guiding strategic business decisions, the application of learning analytics becomes imperative in navigating the educational terrain.
This post takes you on an illuminating journey, exploring the core definition of data-driven learning analytics and dissecting its profound importance in the evolving landscape of L&D tech. From decoding its strategic role in education to addressing prevalent challenges, join us in uncovering insights that will redefine the future of learning.
Table of Contents
The Evolution of Learning Analytics: A Comprehensive Overview

In tracing the historical perspective of learning analytics, we find its roots in the early application of statistical methods in education. The utilization of data to inform educational decisions has evolved from basic record-keeping to more sophisticated models. Early instances, such as the advent of multiple-choice testing in the mid-20th century, marked a departure from subjective evaluation methods.
Technological Advances Shaping Learning Analytics: The Catalysts
- Artificial Intelligence (AI) and Machine Learning (ML): The transformative impact of AI and ML on learning analytics is evident in their ability to analyze vast datasets and provide insights into learner behavior. Predictive analytics, a subset of ML, enables educators to anticipate learner needs and tailor interventions accordingly.
- Data Visualization Technologies: The evolution of learning analytics is closely tied to advancements in data visualization tools. These technologies not only enhance the interpretability of complex data sets but also facilitate effective communication of insights to instructors, administrators, and learners.
Key Milestones in L&D Tech Evolution: Pivotal Moments
- Integration of Learning Management Systems (LMS): The integration of LMS marked a paradigm shift in content delivery and learner management. It streamlined administrative processes, allowing educators to focus on instructional design and personalized learning experiences.
- Emergence of Personalized Learning Approaches: Pivotal in the evolution of L&D tech is the shift towards personalized learning approaches. Adaptive learning platforms, fueled by learning analytics, enable tailored content delivery based on individual learner needs, preferences, and performance.
Ad: PlayAblo’s Enterprise-Grade Micro-Learning platform is built for millennial learners. Micro-Learning, assessments, and gamification features ensure learning outcome measurement and sustained engagement.
Find out more and request a custom demo!
These milestones not only represent technological advancements but also signify a broader shift in educational philosophy, emphasizing learner-centric approaches and the strategic use of data to enhance the overall learning experience.
Current State of Data-Driven Learning Analytics: Insights into Modern Learning Environments
Examining the current landscape of data-centered L&D reveals a multitude of applications in modern learning environments:
- Early Intervention Strategies: Learning analytics enables early identification of struggling trainees, allowing educators to implement timely interventions and support mechanisms.
- Adaptive Learning Paths: Dynamic, data-driven insights facilitate the creation of adaptive learning paths, tailoring educational content to individual learning styles and pace.
- Competency-Based Learning: Learning analytics supports the shift toward competency-based learning, providing real-time feedback on employee mastery of specific skills.
Merger with LMS Platforms
The seamless integration of learning analytics with LMS has become a cornerstone of effective educational technology:
- Enhanced Content Delivery: Learning analytics + LMS optimizes content delivery, ensuring that educational materials align with individual learner needs and performance.
- Administrative Streamlining: LMS integration streamlines administrative tasks, allowing educators to focus on leveraging data insights for instructional improvement.
- Predictive Analytics for Resource Allocation: Institutions leverage predictive analytics within LMS to allocate resources efficiently, forecasting demand for specific courses or additional learning support.
Impact on Learner Engagement and Performance
The influence of numbers and metrics on learner engagement and performance is substantial:
- Personalized Feedback Loops: Learning analytics fosters personalized feedback loops, providing learners with actionable insights into their progress and areas for improvement.
- Gamification Integration: Data, enhanced with gamification elements, enhances engagement, making the learning experience more interactive and motivating.
- Continuous Improvement: Organizations leverage performance data to iteratively enhance training methods, content, and overall learning experiences.
This snapshot of the current state of data-driven Learning Analytics highlights its dynamic applications in modern learning environments, seamless integration with LMS, and the profound impact it has on learner engagement and performance.
Key Components of Effective Data-Driven Learning Analytics

Exploring the foundations of effective learning insights involves diverse data sources and collection methods:
- Multifaceted Data Sources: Effective analytics draw from a diverse range of sources, including learner interactions with online platforms, assessment results, and demographic information.
- Real-time Data Collection: The integration of real-time data collection methods ensures timely access to information, allowing educators to respond promptly to evolving learner needs.
- Qualitative and Quantitative Data: The inclusion of both qualitative and quantitative data provides a holistic understanding of the learning environment, capturing nuances beyond numerical metrics.
Analytical Tools and Technologies
Unlocking meaningful insights relies on sophisticated analytical tools and technologies:
- Predictive Analytics: The utilization of predictive analytics forecasts future trends, enabling educators to proactively address challenges and optimize learning pathways.
- Machine Learning Algorithms: Integrating machine learning algorithms enhances the analysis of complex datasets, identifying patterns and trends that may not be apparent through traditional methods.
- Visualization Techniques: Effective communication of insights is facilitated by visualization techniques, translating complex data into accessible and actionable information for educators.
Importance of Data Privacy and Security
Ensuring the integrity and ethical use of learning data underscores the importance of data privacy and security:
- Robust Privacy Measures: Implementing robust privacy measures safeguards sensitive learner information, fostering trust among students, educators, and stakeholders.
- Compliance with Regulations: Adhering to data protection regulations ensures ethical and legal use of learning data, maintaining the integrity of the learning analytics process.
- Transparent Data Governance: Transparent data governance frameworks establish clear guidelines for the ethical collection, storage, and utilization of learning data, emphasizing accountability and responsibility.
By understanding and optimizing these key components, educators can harness actionable insights without compromising learner privacy and security.
Learning and Development Technology Trends Driven by Data Analytics

As we gaze into the future of L&D trends, the integration of AI and ML stands as a pivotal trend:
- Adaptive Learning Systems: AI and ML integration will propel the development of adaptive learning systems, tailoring educational content dynamically based on individual learner needs.
- Cognitive Computing: Future applications include cognitive computing, where AI algorithms analyze intricate data sets to understand learner behaviors, preferences, and challenges, shaping more responsive and intuitive learning environments.
- Automated Feedback Mechanisms: The integration of AI fosters automated feedback mechanisms, providing instant insights into learner progress and areas for improvement.
Personalized Learning Journeys
The future of L&D tech is destined to be marked by an emphasis on personalized learning journeys:
- AI-Driven Personalization: Advanced personalization, driven by AI algorithms, will create tailored learning experiences that cater to each learner’s unique strengths, weaknesses, and preferences.
- Adaptive Content Delivery: Personalized learning journeys will prioritize adaptive content delivery, ensuring that educational materials align with individual learning styles, and fostering more effective and engaging learning experiences.
- Holistic Learner Profiles: A holistic approach to personalized learning will involve the creation of comprehensive learner profiles, incorporating data beyond academic performance to encompass socio-emotional aspects and extracurricular interests.
Predictive Analytics for Skill Development
The future landscape of L&D tech will witness the widespread adoption of predictive analytics to drive skill development:
- Anticipatory Skill Mapping: Predictive analytics will enable anticipatory skill mapping, identifying future skill demands in the job market and guiding the development of relevant educational programs.
- Continuous Learning Paths: Educational platforms will leverage predictive analytics to design continuous learning paths, aligning with the evolving demands of industries and ensuring learners are equipped with cutting-edge skills.
- Dynamic Skill Recommendations: Personalized skill development will be enhanced by dynamic recommendations generated through predictive analytics, empowering learners to make informed decisions about their learning journey.
Ad: PlayAblo’s Enterprise-Grade Micro-Learning platform is built for millennial learners. Micro-Learning, assessments, and gamification features ensure learning outcome measurement and sustained engagement.
Find out more and request a custom demo!
Best Practices for Implementing Data-Driven Learning Analytics
Crafting a successful implementation strategy involves meticulous alignment of analytics with learning objectives:
- Clarify Learning Goals: Begin by clearly defining learning objectives, ensuring that the analytics focus on key outcomes aligned with educational priorities.
- Customized Metrics: Tailor metrics and data collection methods to directly reflect progress towards learning objectives, fostering a more nuanced and insightful understanding of student performance.
- Regular Goal Assessments: Periodically assess the alignment between analytics and learning objectives, adapting strategies to evolving educational priorities and student needs.
Involving Stakeholders and Creating a Data-Driven Culture
A holistic implementation approach necessitates the active involvement of stakeholders and the cultivation of a data-driven culture:
- Collaborative Decision-Making: Engage educators, administrators, and students in collaborative decision-making processes, ensuring that the insights generated by analytics are contextualized and effectively utilized.
- Training and Education: Promote a data-driven culture through comprehensive training programs, empowering stakeholders with the knowledge and skills needed to interpret and apply learning analytics effectively.
- Transparent Communication: Foster transparent communication about the purpose and benefits of learning analytics, cultivating a shared understanding of its role in enhancing educational outcomes.
Continuous Monitoring and Adaptation Strategies
The journey toward effective learning analytics implementation is an iterative process that demands continuous monitoring and adaptation:
- Real-Time Monitoring: Implement real-time monitoring systems to promptly identify trends, challenges, and opportunities, allowing for agile responses and interventions.
- Feedback Loops: Establish robust feedback loops involving educators and learners, creating mechanisms for continuous improvement based on insights gleaned from analytics.
- Adaptation to Technological Advances: Stay abreast of technological advancements and regularly update analytical tools and methodologies to harness the full potential of emerging innovations.
Ethical Considerations in Data-Driven Learning Analytics
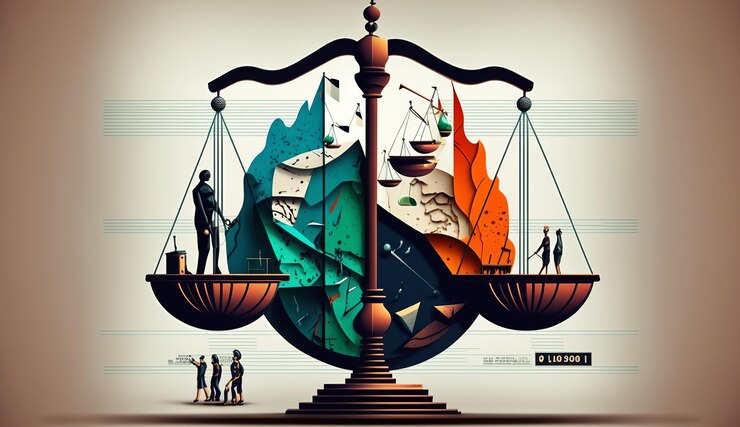
Privacy Concerns and User Consent
Respecting privacy and obtaining user consent are paramount in ethical data-driven learning analytics practices:
- Informed Consent: Prioritize transparent communication with users, ensuring they are fully informed about the data collected, how it will be used, and any potential implications.
- Data Anonymization: Implement robust data anonymization techniques to protect the identity of individuals, minimizing the risk of unintended disclosure.
- User Control: Empower users with control over their data, allowing them to decide the extent to which their information is utilized and shared.
Ensuring Fairness and Avoiding Bias
The ethical use of learning analytics requires a commitment to fairness and the avoidance of bias:
- Fair Algorithmic Models: Regularly assess and refine algorithms to ensure they are fair, unbiased, and do not disproportionately impact specific demographics or groups.
- Diversity in Data Representation: Strive for diverse representation in the data used, avoiding underrepresentation or overrepresentation of specific groups to prevent skewed results.
- Regular Audits: Conduct regular audits of algorithms and data sources to identify and rectify potential biases, promoting equal opportunities for all learners.
Transparency in Data Usage
Maintaining transparency in how data is used is fundamental to building trust and upholding ethical standards:
- Clear Communication: Clearly communicate the purposes for which data is collected and how it will be utilized, fostering an understanding among users.
- Accessible Policies: Make privacy policies and terms of use easily accessible and understandable, enabling users to make informed decisions about their data.
- Openness about Outcomes: Be transparent about the outcomes and insights generated through L&D analytics, ensuring stakeholders understand the implications and limitations of the data-driven processes.
Case Study: VitaTech.Org Leveraging Data-Driven Learning Analytics
A. Hypothetical Example of VitaTech.Org | B. Measurable Improvements in Employee Performance and Skill Development | C. Lessons Learned from Implementations |
---|---|---|
VitaTech.Org, a technology firm, integrates learning analytics. | Employee performance improves by 25% based on real-time feedback. | 1. Clear Objectives: Define clear learning objectives aligning with organizational goals. |
Learning analytics track individual progress and preferences. | Skill development time reduced by 30% with personalized learning paths. | 2. Continuous Monitoring: Establish real-time monitoring systems for agile intervention. |
Customized learning paths enhance employee engagement. | Employee satisfaction increases by 20%, reflecting personalized experiences. | 3. Stakeholder Involvement: Actively involve stakeholders in decision-making processes. |
VitaTech.Org sees a 15% increase in overall productivity. | Skill development time is reduced by 30% with personalized learning paths. | 4. Privacy Safeguards: Prioritize robust data anonymization and user consent mechanisms. |
5. Fair Algorithmic Models: Regularly assess algorithms to ensure fairness and avoid bias. | ||
6. Transparency in Communication: Maintain clear communication about data usage and outcomes. |
This hypothetical case study illustrates how VitaTech.Org leverages data-driven analytics to achieve measurable improvements in employee performance and skill development. The lessons learned emphasize the importance of clear objectives, continuous monitoring, stakeholder involvement, privacy safeguards, fair algorithms, and transparent communication.
Conclusion
In the ever-evolving landscape of L&D technology, data and analytics emerge as a pivotal force. From its historical roots to current applications and future trends, this exploration delves into the transformative potential of analytics in education.
Ethical considerations, best practices, and a compelling case study underscore the intricate tapestry of this tech-driven educational journey. As organizations navigate the future, the integration of learning analytics stands as an indispensable compass, guiding the way to enhanced educational outcomes and a more adaptive, empowered workforce.
Ad: PlayAblo’s Enterprise-Grade Micro-Learning platform is built for millennial learners. Micro-Learning, assessments, and gamification features ensure learning outcome measurement and sustained engagement.
Find out more and request a custom demo!
Comments are closed, but trackbacks and pingbacks are open.